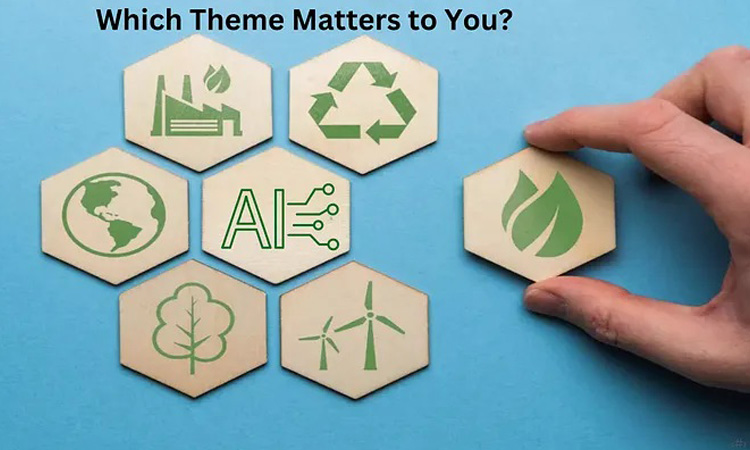
Thematic Investing: Catching Themes with AI
July 3, 2023
Due to recent advances in NLP, algorithms are capable of ingesting raw textual data about stocks from various sources, revolutionizing trading. But how do you convert complex conversations about various topics to investment themes? Let’s look at how “thinking in themes” can be a powerful solution
Stock trading strategies have been in existence for a considerable duration. While the origins of systematic quantitative trading can be traced back approximately 50 years to the 1970s, where technical trading emerged, some form of trading has persisted since ancient times. Thales of Miletus, for instance, reputedly utilized his knowledge of weather and agriculture to make profitable bets on related outcomes (think commodities trading). Trading necessitates knowledge. Possessing more accurate information about the present compared to others or the ability to make predictions, albeit imperfect ones, can be advantageous [2].
Until about ten years ago, traders primarily relied on quantitative data, such as numbers, as inputs for their trading strategies in their pursuit of profit. However, during the 2010s, NLP (Natural Language Processing) techniques gained increasing acceptance among quantitative researchers and traders. Algorithms became capable of ingesting raw textual data about stocks from various sources like news, social media, blogs, and audio, generating tradable signals in the process.
Sentiment Analysis
One of the earliest NLP signals to be traded was sentiment analysis. Researchers and practitioners [3] devised methods to score keywords mentioned in textual data related to stocks, aiming to discern market sentiment concerning those stocks. The underlying idea was that if news conveyed a positive sentiment about a stock, it was likely to be on an upward trajectory or poised for significant growth. While several approaches to measuring sentiment in text existed, Loughran and MacDonald developed a specific list of keywords for measuring sentiment within the financial context. More sophisticated versions of sentiment analysis can gauge the sentiment conveyed in Federal Reserve speeches and apply it to various instruments.
From Sentiment to Themes: Unraveling the Factors
While sentiment analysis uncovers one of the signals concealed in textual data, numerous other types of signals can be linked to specific themes. The constant stream of news contains diverse information. A single news text published within an hour on any given day can reveal market sentiment, controversies, a company’s commitment to addressing climate change or biodiversity concerns, or how geopolitical factors affect a company, among others. In fact, market sentiment may be the ultimate result of an underlying current of themes [Figure 1].

Figure 1: A series of related but loosely independent themes can come together to result in a final sentiment.
Embracing a Thematic Approach
Different users of these signals find different themes relevant. An investor interested in green investments will seek signals that indicate shifts in sentiment regarding electric vehicles, solar power, EV batteries, and related topics. On the other hand, a human rights watchdog will focus on any information pertaining to human rights violations. Figure 2 illustrates different themes extracted from the news flow about Tesla over the same time period. It is also possible for the same news flow to be relevant to multiple themes.
The environment relevant news (in green) in Figure 2 is relevant from the market sentiment angle as well since it involves changes to Tesla’s business model. Rather than solely relying on a handful of scores, such as a sentiment score, it is crucial to adopt a multifaceted approach that incorporates multiple themes.
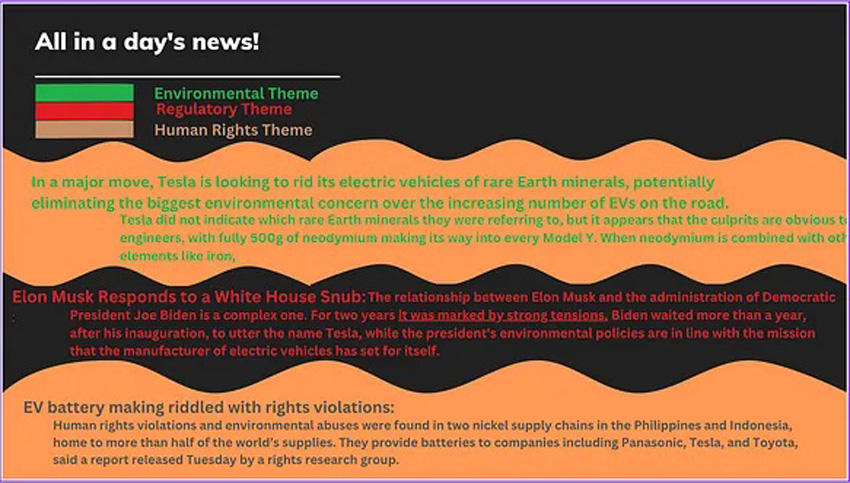
Figure 2: News flow for Tesla on the same day covering multiple themes with different implications. Different users will be interested in different such themes.
Better AI = better theme discovery
But how to extract themes accurately and effectively? Let us see how NLP models have evolved over time to give more accurate results. One could take a simple keyword based approach and look for any information item on the web that contains the keyword. For concreteness, let’s say we are looking for information on the web related to China’s GDP and use the keyword “China GDP”. The results from this approach will range from very relevant to irrelevant information. Irrelevant information will be contextually about something else, but will just happen to have the specific keyword. See for example, the results obtained from this exercise for China’s GDP [Figure 3]. Result 1 is highly relevant, but the second result is actually about a machine learning technical paper that discusses using AI methods to forecast China’s GDP. If we want only that information which is relevant for a economic or political analysis of China’s economy, chances are that the second result will be entirely irrelevant for us.
One can, of course, make keywords more complex. But that will make the keyword so specific that approach will lose the sight of the forest for the trees. In technical terms, such very specific keywords will lead to over-fitting and will do very poorly in practice. The solution, of course, is to make the NLP models context aware. This is where the recent advances in GPT has dazzled the world. These new generation of models appear to be context aware in various settings and can handle multiple use cases.
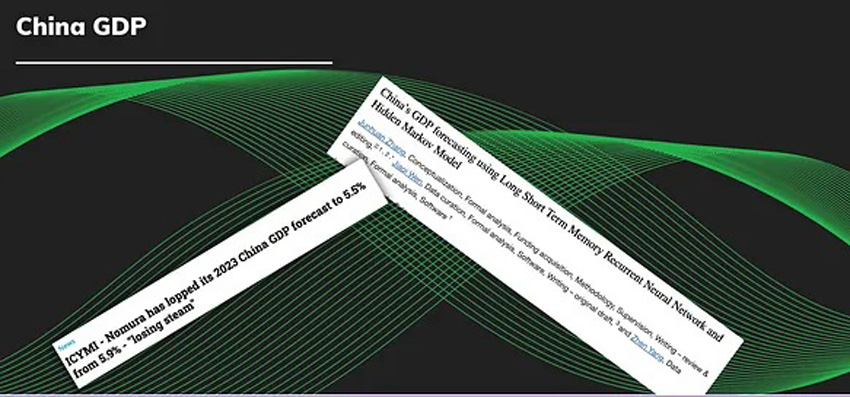
Figure 3: Two different results from google search query for the keyword “China GDP” with very different contexts.
Whither Water?
Consider the theme “Water”. Figure 4 provides an entertaining example of why a context-aware approach is necessary. Searching for news relevant to water-related environmental issues yields two results. One result discusses extreme climate conditions in the Southern United States in December 2022, while the other pertains to the Disney movie, Avatar: The Way of the Water, also released in December 2022. The discussion of the Avatar movie explores its environmental undertones. Therefore, the NLP model must be particularly adept at determining whether to include this text when the objective is to collect news items about water in an environmental context.
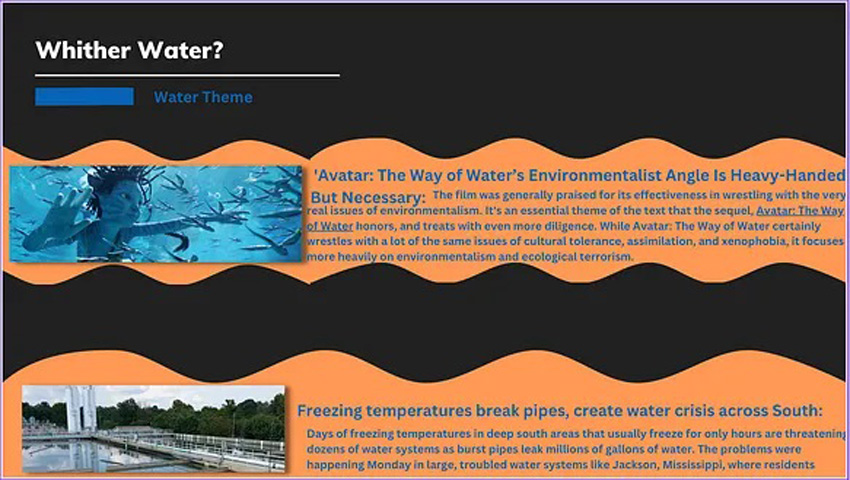
Figure 4: Two different results from google search query for the keyword “Water” for the same time period of December 2022. Both results have an environment/climate undertone.
Multilingual AI: Themes Across Multiple Languages
To make our discussion even more intriguing, let us consider what happens when we move beyond the English language. To keep things simple, let us continue with the theme of “Water” and the time period of December 2022. Suppose we search for news items in China, specifically in Chinese, that discuss water. The Chinese character for water is 水. A range of results appears. Figure 5 showcases a few examples. Since Chinese employs a character-based writing system, it presents a greater challenge for machine learning techniques compared to English. As seen in Figure 5, one of the results concerns the US-based hedge fund, Bridgewater Associates, and its portfolio returns in December 2022.
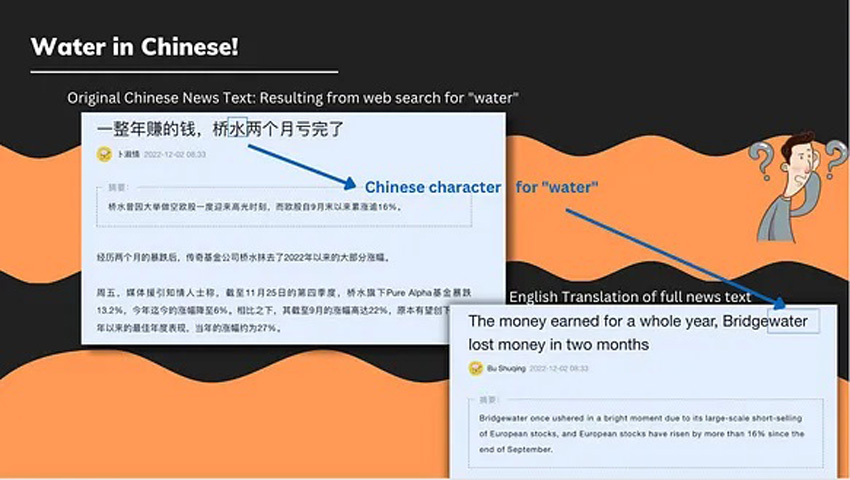
Figure 5: Two different results from google search query for the keyword “Water” for the same time period of December 2022. Both results have an environment/climate undertone.
EMAlpha’s Multilingual Thematic NLP
EMAlpha embarked on research in 2018 with the aim of developing NLP solutions capable of generating accurate thematic results in multilingual settings. Currently, EMAlpha is able to generate actionable scores for trends and risks across 80+ themes, including market sentiment, geopolitical risk, business risk, and ESG (Environmental, Social, and Governance) risk, covering 50+ countries and multiple languages.
In addition, EMAlpha offers GPT models specifically trained on emerging markets data, encompassing countries such as China, India, Brazil, Russia, and more. This enables clients to create knowledge discovery and information retrieval software, facilitating quick access to relevant and actionable global data.
EMAlpha’s comprehensive suite of solutions, ranging from data to customized LLM-based software solutions, presents an ideal solution for companies with a global presence. These companies require AI-driven solutions that go beyond news and information originating from a few English-speaking countries, and instead, address the need for handling global information across diverse countries, cultures, and languages. A globally connected world needs AI solutions that are truly global in nature.